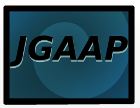
The role that software plays in stylistic analysis of text is perhaps less surprising to high school and college students than to the general public. The former must submit essays they write to style analysis performed by software which looks for plagiarism and sometimes also makes quality assessments.
In the recent outing of J.K. Rowling as the writer behind the pen name Robert Galbraith, it was mentioned that software had been used to analyze the text of the Galbraith novel. There exists a family of software used by academics for “authorship attribution,” e.g., to discover, for example, whether a recently discovered manuscript was a missing chapter of Don Quijote (a fabricated example). One of these applications is JGAAP, for Java Graphical Authorship Attribution Program. The JGAAP wiki page explains the project as
. . . Java-based, modular, program for textual analysis, text categorization, and authorship attribution i.e. stylometry / textometry. JGAAP is intended to tackle two different problems, firstly to allow people unfamiliar with machine learning and quantitative analysis the ability to use cutting edge techniques on their text based stylometry / textometry problems, and secondly to act as a framework for testing and comparing the effectiveness of different analytic techniques’ performance on text analysis quickly and easily. JGAAP is developed by the Evaluating Variation in Language Laboratory (EVL Lab) and released under the AGPLv3.
How this was accomplished was explained by one of two academic investigators credited with the analysis (along with some suspicions by reports at the Sunday Times) at . Patrick Juola, in the blog Language Log. Juola refers to this subdiscipline as “forensic stylography.”
A one-paragraph extract from Juola’s blog post follows. Note that, in the usual sense of the word, the analysis doesn’t look directly at “meaning.”
The heart of this analysis, of course, is in the details of the word “compared.” Compared what, specifically, and how, specifically. I actually ran four separate types of analyses focusing on four different linguistic variables. While anything can in theory be an informative variable, my work focuses on variables that are easy to compute and that generate a lot of data from a given passage of language. One variable that I used, for example, is the distribution of word lengths. Each novel has a lot of words, each word has a length, and so one can get a robust vector of <X>% of the words in this document have exactly <Y> letters. Using a distance formula (for the mathematically minded, I used the normalized cosine distance formula instead of the more traditional Euclidean distance you remember from high school), I was able to get a measurement of similarity, with 0.0 being identity and progressively higher numbers being greater dissimilarity.